As the AI market matures from training to inference workloads, the time for partners to capitalize on inference is now. If you’re still coming up to speed on AI terminology and go-to-market strategies, our friends at Cloudflare covered the differences between “Training” and “Inference” in a great blog. Here are the Cliff’s Notes from the post:
- Training is the first phase for an AI model. Training may involve a process of trial and error, a process of showing the model examples of the desired inputs and outputs, or both.
- Inference is the process that follows AI training. The better trained a model is, and the more fine-tuned it is, the better its inferences will be — although they are never guaranteed to be perfect.
More simply, training costs money while inference makes money.
But what does this mean for resellers, integrators, and their customers? Let’s discuss.
The most important thing to consider is that training and inference require vastly different types of infrastructure. Training, for example, leverages very fast GPUs that consume incredible amounts of power (750w-1kw each), making it very difficult to optimize the cost of these environments, measured by “tokens/dollar” or “tokens/watt.” For background on the lingo, a “token” is a unit of measurement in AI, such as a word being read or an image being processed—in other words, “how much information can my model review for the money I am investing.”
As the last several months have shown, only the largest companies in the market (OpenAI, Tesla, Google, Meta, etc.) have the resources to perform training at scale, while mere mortals simply don’t have the power (literally and figuratively) to compete. Mark Zuckerberg recently highlighted this during an interview with Dwarkesh Patel, saying, "Energy, not compute, will be the #1 bottleneck to AI progress."
So, if participating in training projects is off the table for most customers, does that mean resellers and integrators are on the outside looking in for AI revenue? No. Why? Because inference will soon be bigger than training. In fact, as shown in the graph below, computing needs for training applications are expected to level off in 2024/2025 while inference starts to take off, culminating in inference consuming 90% of AI computing needs by 2030.
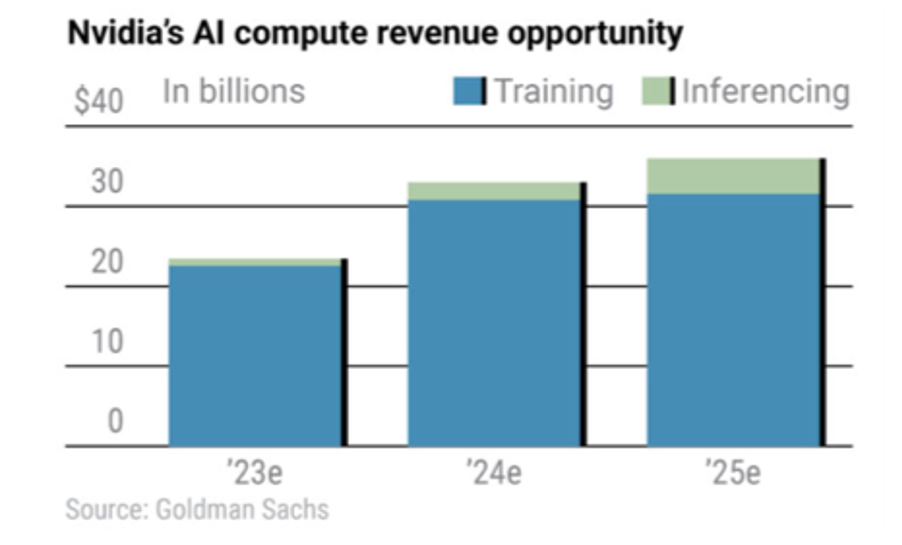
How do we expect this all to play out? Well, when customers are interested in training a new model, they will use cloud infrastructure, leveraging the big players who can power such environments (keep an eye on the hyper-scalers using modular nuclear power). Less power-hungry, on-prem or edge data center infrastructure will be leveraged to move the model into production (i.e., inference).
End result? OEM and channel partners need to start having inference conversations now. Optimizing for inference—and building out the infrastructure to do it efficiently—is the next big thing in AI. This will require open standards with the ability to work among vendors and devices to meet the requirements of these individual deployments. They will differ vastly depending on the specific function, but energy efficiency and effective footprint management will be key as AI moves from buzz to widescale production over the next few years. The most successful providers will be nimble enough to identify what technology works best with the task and set their customers up to demonstrate early wins, gaining greater buy-in and setting them up for long-term success.